
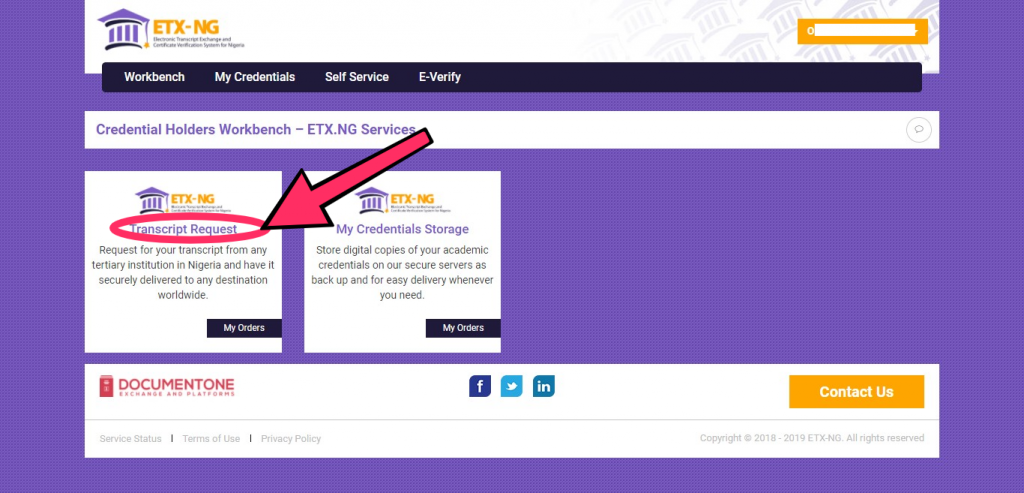
- #Good annotations v bad annotations students how to
- #Good annotations v bad annotations students full
While this study looks at human annotations, it's important to note that humans are often trained using datasets that are not comprised of relevant data. This shows that using a computer to make image annotations can be very inaccurate. One study compared the ability of humans to label an image as containing a horse against a computer's ability to label the same image as containing a horse, with the computer achieving an accuracy rate of 56% (humans had an accuracy rate of 92%).
#Good annotations v bad annotations students full
Many researchers have published research about this, including datasets that are full of bad or nonsense annotations. We want to do anything we can to make sure that our training data is actually useful and accurate.īad annotations are more common than you may think.

Why Do We Need To Correct Bad Annotations?īeing able to identify and correct bad annotations is important in several important areas: making data more accurate, improving performance of the model, and updating the overall quality of the annotation dataset. This could cause problems for machine learning if an algorithm thinks that it's recognizing something that's supposed to be in the picture. An example of this is when humans annotate a picture of a dog, but the annotated image contains a dog with eyes in its head (a common caricature). However, the problem with image annotations is that many are not accurate or complete. Image annotations are useful because they provide useful information to a machine learning task. In fact, it's been shown by many researchers that having accurate image annotations can significantly improve machine learning results by providing large sets of training data or improving the accuracy of the data when "teaching" algorithms to recognize patterns better. By not having good image annotations, you're missing out on a lot of benefits that image annotations can provide.
#Good annotations v bad annotations students how to
Through a process of collaborative interactive annotation, HyLighter promotes understanding of text, develops learning how to learn skills, improves instructional quality, and increases productivity in document-centered workgroups.Image annotations are commonly used in computer vision and other AI tasks, but they don't get much attention. In effect, HyLighter makes the thinking of readers that is ordinarily hidden, become "transparent" and easily accessible for self-reflection and sharing with others. HyLighter builds on and goes beyond existing hypertext annotation technology with the addition of a new and unique feature: the facility to aggregate or combine annotations from multiple readers and generate composite displays. This includes, for example, the capacity to highlight important text and add remarks to web pages. Online annotation systems allow readers to mark up electronic reading material in ways similar to paper and share annotations with other people over a computer network. This paper describes a unique online annotation system, referred to as HyLighter, and summarizes results of evaluation efforts to date. Findings from the gathered literature were synthesized to provide recommendations for using SA technology in educational settings.
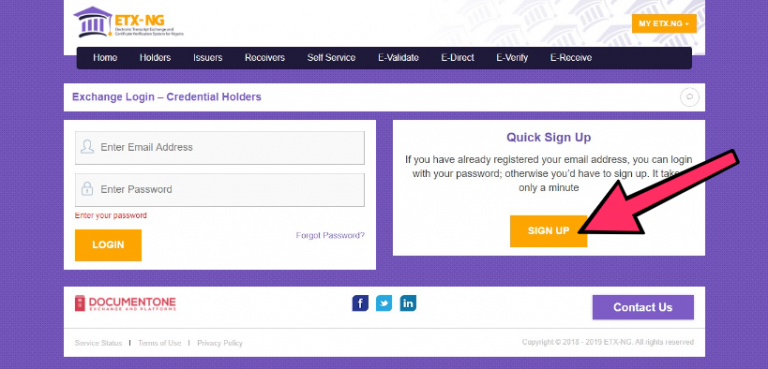
The SA empirical research has provided some evidence regarding the potential effectiveness of integrating social annotation tools into learning activities. Among the included studies were eight experimental or quasi-experimental studies and eight evaluation/survey studies. Out of more than 90 articles that were initially found, only 16 studies met the inclusion criteria. The literature review has aimed at presenting a comprehensive list of SA empirical studies not limited to a particular research method or study domain. As such, the research focusing on this technology is still very limited. SA technology is an emerging educational technology that has not yet been extensively used and examined in education. This paper presents a literature review of empirical research related to the use and effect of online social annotation (SA) tools in higher education settings.
